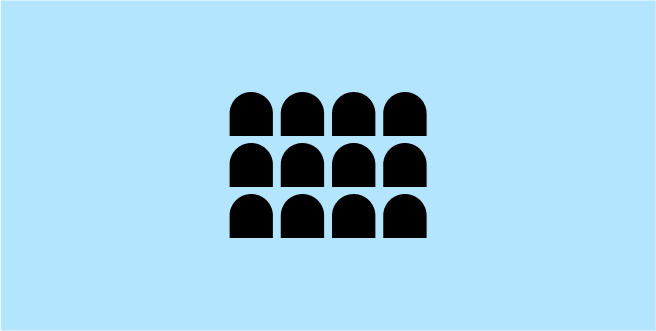
Challenges & future trends in data product development
Data quality and integrity are some of the most common challenges faced during the development of data products.
It’s important to ensure that the data is accurate, consistent, and reliable since any issues with these factors can adversely affect the effectiveness of the data product.
In fact, data quality is likely to be the most vital factor to consider since a significant part of the data product’s value depends on it.
The key to overcoming these challenges lies in thorough data cleaning and, more critically, in meticulous verification and enhancement of data collection processes.
Staying ahead while maintaining safety & privacy
Staying at the forefront of technology, especially with data sharing and integrating machine learning, deep learning, and generative AI, presents unique challenges in maintaining stringent data security and privacy.
This concern is central to the entire integration process.
Developers must navigate a complex landscape of legal and ethical requirements to ensure that data products not only innovate but also rigorously protect user data. It’s a delicate balance, crucial for sustaining trust and compliance.
The duality of data adoption & scalability
In a market where anyone with data and software knowledge can create a data product, standing out and securing user engagement is a formidable task. Successfully crossing this adoption barrier then leads to another hurdle: scalability and maintenance.
As data products expand, scaling them efficiently without compromising performance becomes an increasingly complex endeavor, requiring strategic planning and robust infrastructure.
Data democratization vs. sovereignty
In the evolving data landscape, a dynamic tension is emerging between the trends of democratization and sovereignty. As industries gravitate towards specialization, companies are shifting their focus to core activities, paving the way for data democratization.
This movement aims to make data more accessible within organizations, empowering a broader range of users.
In contrast, the trend of sovereignty is gaining traction, emphasizing data independence and strict governance in response to geopolitical pressures. This contrast highlights the growing complexity of balancing open access to data with the need for stringent control and ethical practices.
Valuable data is only of high quality
As predicted, there will be a growing demand for high-quality data in the upcoming years.
Generative AI is already widely used and is expected to continue growing, and the success of generative AI depends on the quantity and surely more on the quality of data it has access to, which will make high-quality data even more valuable in the future.
The importance of frugal data consumption
Ecology has become a worldwide concern, and we are becoming increasingly aware that data and computation are resource-intensive, not only in terms of money but also in energy consumption and environmental impact.
Therefore, we can imagine a day when data authorities will establish a policy forcing companies to adopt data frugality, i.e., collecting and using only the strictly necessary data.
The most effective way to overcome these challenges is by having a well-articulated and diverse team.
Data Scientists, Engineers, salespeople, and business stakeholders bring different perspectives and expertise to the table. They help in understanding data, shaping technology, driving revenue, and providing context and goals.
This collaborative approach, blending diverse skills and viewpoints, is essential in crafting data products that are not only innovative but also resonate with the needs of a diverse and dynamic market.
Conclusion
In conclusion, developing data products presents an array of challenges and future trends that require a multifaceted approach. Ensuring data quality and integrity is paramount, as these factors are foundational to the effectiveness of any data product.
Overcoming these challenges involves robust data cleaning and rigorous enhancement of data collection processes.
Looking ahead, the demand for high-quality data will continue to rise, driven by the growth of generative AI. This underscores the importance of data frugality, where only essential data is collected and used to mitigate environmental impacts.
The key to navigating these complexities lies in fostering a collaborative and diverse team of data scientists, engineers, salespeople, and business stakeholders.