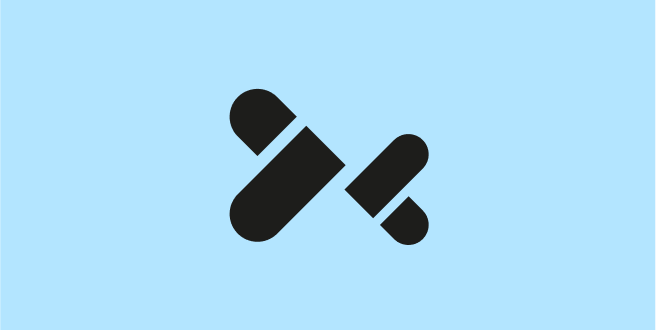
Data governance & observability: 3 steps to combined value
Data governance and data observability are interdependent. While governance establishes the rules and standards for data management, data observability ensures those rules are being followed in real-time.
Together, they create a feedback loop that reinforces data trust and AI readiness.
This article will discuss the basics of data governance and observability, discuss the best options to implement both in your organization, and how DataGalaxy and Bigeye offer a best-of-breed platform for creating a winning strategy.
The interplay of data governance & observability
Though similar, data governance and observability have a few key differences.
Data governance defined
Data governance refers to the process of managing the availability, usability, integrity, and security of the data in enterprise systems. Data governance covers all the rules and methods that ensure an organization's data structure, protection, and management.
There is no one-size-fits-all approach to data governance. The most critical first step in creating a lasting data governance strategy is to define and thoroughly map out your objectives.
Good data governance ensures that the data you and your teams use is correct, consistent, and accessible to the right people in the company.
What is data observability?
Data observability is the ability to fully understand, monitor, and troubleshoot the health and quality of data across your entire system.
By tracking key metrics such as data freshness, accuracy, completeness, and consistency, teams can quickly detect issues like broken pipelines, unexpected changes, or data downtime before they cause significant problems.
Strong data observability is essential for maintaining trust, improving data quality, and ensuring that governance efforts are built on reliable, high-performing data.
Data observability & governance: Working together for data success
Data observability and data governance go hand in hand because both are essential for ensuring that an organization's data is reliable, secure, and trustworthy.
While data governance sets the rules, policies, and responsibilities for managing data, data observability provides the real-time visibility needed to monitor whether those rules are being followed and if the data remains healthy over time.
Observability acts as an early warning system, quickly surfacing issues like data quality problems, pipeline failures, or unauthorized changes that governance policies are designed to prevent.
Governance defines the standards, and observability ensures that those standards are continuously met, helping organizations make better decisions and maintain compliance with confidence.
Governance ensures accountability
Data governance tools monitor compliance with governance policies, such as data lineage and access controls
Observability provides insights
Data observability can inform governance decisions by highlighting areas where policies need refinement or additional enforcement
Data governance & observability implementation options
There are several implementation options for data governance and data observability:
1. Homegrown solutions: Using spreadsheets & internal resources
Pros:
- Cost-effective start: Homegrown solutions such as spreadsheets, Python scripts, or internal databases are often inexpensive or even free to implement initially.
- Customizable: Organizations can tailor the system to their specific needs and processes, allowing for quick adaptations in early stages
Cons:
- Scalability challenges: As data grows and the organization expands, these solutions struggle to scale efficiently, often leading to inefficiencies and data inconsistencies.
- Fragmented ownership & documentation: Managing data definitions, quality, and lineage becomes cumbersome, making it difficult to track ownership and ensure compliance.
Key considerations:
- Resource-intensive: Building and maintaining a homegrown solution requires a substantial amount of time and effort from engineering, data, and product teams. Over time, this can shift from a cost-effective strategy to an expensive burden.
- Limited collaboration: Lack of standardized processes can hinder collaboration across departments and increase the risk of errors or misunderstandings.
Example technologies:
- Uber, Airbnb, and Netflix started with homegrown solutions that evolved as the organizations scaled. However, they eventually shifted to more robust third-party platforms to manage data governance and data observability at scale.
2. All-in-one platform: A single vendor solution
Pros:
- Simplified procurement: Working with a single vendor for all data governance and data observability needs reduces complexity and streamlines the purchasing and implementation process.
- Seamless integration: Components from the same vendor typically work together more easily, reducing the likelihood of integration issues between different systems or tools.
Cons:
- Solutions may be spread too thin: A single vendor may not offer best-in-class capabilities across all aspects of data governance, leading to compromises in functionality or performance.
- Inconsistent user interface: Vendors providing an all-in-one solution may not have the resources or focus to maintain a consistently user-friendly interface across all components.
- Limited negotiation power: With all tools from one vendor, there’s less room to negotiate on pricing or customize the solution for your specific needs.
Key considerations:
- Legacy systems integration: The implementation of an all-in-one platform may be complicated by existing infrastructure or legacy systems, making integration more difficult than anticipated.
- Resource constraints: Depending on the scale of your organization and data governance needs, resource constraints could limit the effectiveness of an all-in-one solution.
Example technologies:
- IBM, Informatica, and Microsoft offer comprehensive data governance and data observability platforms that aim to provide a one-stop solution for organizations.
3. Best-of-breed integration: Integrating specialized vendors
Pros:
- Comprehensive, tailored solution: By selecting specialized vendors for different components of data governance and data observability, you can ensure that each aspect of the solution is best-in-class.
- Expertise from specialized vendors: Each vendor brings focused knowledge and expertise to their specific area, providing a more in-depth and robust solution than an all-in-one platform might offer.
Cons:
- Integration complexity: Integrating multiple specialized vendors requires seamless data flow between different systems and careful management to ensure compatibility, which can lead to technical and operational challenges.
- Inconsistent user experience: With multiple vendors involved, it can be challenging to maintain a consistent user interface across tools, leading to potential frustration for end users.
Key considerations:
- Vendor alignment: It’s crucial to understand how well your selected vendors can work together, as partnerships and integrations between tools play a critical role in the success of a best-of-breed strategy.
- Complex management: While each tool excels in its domain, managing multiple vendors can be resource-intensive, and integration between systems must be actively monitored to prevent issues from arising.
Example Technologies:
- DataGalaxy and Bigeye are examples of specialized vendors that, when integrated, offer a powerful solution for both data governance and data observability, ensuring comprehensive coverage across the organization’s needs.
DataGalaxy & Bigeye: Combined value
DataGalaxy and Bigeye together create the ideal best-of-breed, all-in-one platform for organizations looking to master both data governance and data observability.
DataGalaxy offers a robust, collaborative solution for mapping, managing, and governing data assets, helping teams ensure data is well-organized, compliant, and aligned with business goals.
Bigeye complements this by delivering automated data observability, enabling real-time monitoring of data quality, freshness, and reliability across every system.
By combining DataGalaxy’s intuitive governance capabilities with Bigeye’s deep observability insights, organizations gain a comprehensive, end-to-end view of their data ecosystem.
This integrated approach empowers teams to not only define and enforce data standards but also to detect and address issues before they impact operations, building a foundation of trust, transparency, and operational excellence across the entire data landscape.
The combined value of DataGalaxy and Bigeye includes:
Data governance:
DataGalaxy provides comprehensive data cataloging and lineage.
Trust:
The combined approach builds confidence in data for AI initiatives.
Observability:
Bigeye ensures complete visibility of pipeline health and data quality.
Value:
Seamless connection between platforms creates an end-to-end solution.
DataGalaxy x Bigeye product highlights
Key product highlights of DataGalaxy and Bigeye's data governance and observability platform include:
- Tracking data quality scores on all business metrics
- Automation with bi-directional sync between DataGalaxy and Bigeye's data observability
- Alerts to notify teams about data pipeline or quality issues
- Column-level history to audit data quality
- Data quality rules library to create standards in plain language
- A centralized place to manage data governance & quality remediation
Conclusion
Building AI readiness and data trust requires a strategic approach to data governance and data observability.
By implementing these strategies, organizations can ensure data reliability, improve operational efficiency, and unlock the full potential of AI.
The combined value of best-of-breed solutions like DataGalaxy and Bigeye provides a comprehensive foundation for achieving these goals.