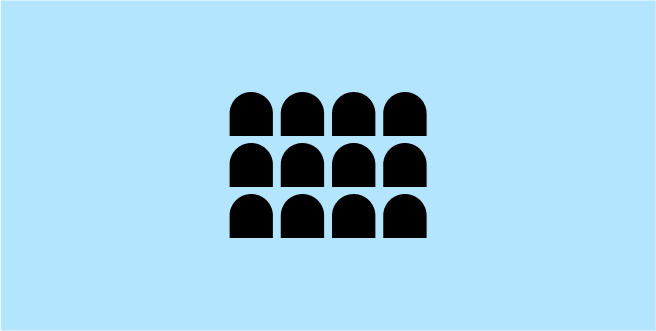
Data lake vs. data warehouse: What’s the difference?
While both serve as critical data management components, data lakes and warehouses offer distinct approaches to storing, processing, and utilizing data.
Understanding the differences between these two systems is crucial for organizations to optimize their data strategies and meet their unique needs.
Data lakes & data warehouses defined
In data management, two prominent players stand out: Data lakes and data warehouses. A data lake is like an expansive reservoir where data flows in its rawest form without predetermined structures or schemas.
Imagine a data lake as a vast, untouched wilderness where data flows in its purest form without the constraints of predefined structures or schemas. This flexibility allows organizations to capture diverse data sources, making it an invaluable resource for data professionals, IT managers, and business analysts.
Furthermore, data lakes embrace the concept of data-on-demand. Data professionals using DataGalaxy, for example, can explore and analyze data in its raw form without extensive preprocessing. This flexibility is essential when dealing with ever-evolving data sources, enabling quick adaptation to changing data needs.
Conversely, a data warehouse resembles an organized library, meticulously categorizing structured data with predefined schemas. Data is carefully transformed and structured before entering.
Structured data in DataGalaxy's data warehouse is designed for efficient querying, reporting, and analysis. Using this tool, Business Analysts can swiftly extract meaningful insights from well-organized datasets, supporting informed decision-making and strategic planning.
Data storage & schema
In a traditional data warehouse, data storage resembles a carefully organized library, where each piece of data has its place in predefined tables and columns.
This structured approach ensures data consistency and integrity, making it suitable for well-defined and structured data, such as financial records or customer profiles. Data schema, if required, can be applied on the fly during data processing. Data is structured only when necessary, saving time and resources.
Data processing & transformation
Data processing encompasses various techniques, including cleansing, aggregation, and enrichment. Data professionals can apply transformations to shape data for reporting, modeling, and visualization purposes.
Whether dealing with terabytes or petabytes of data, organizations can rely on a data lake for scalability and processing capabilities. This is crucial in today's data-rich environment, where the volume of data continues to grow exponentially.
Furthermore, data transformation plays a key role in data analytics. Data professionals can create data pipelines to automate the transformation process, making it efficient and repeatable. This automation accelerates the time-to-insight, allowing organizations to make informed decisions more quickly.
Query performance & use cases
One of the key use cases of data lakes is real-time analytics. Organizations can monitor data streams and receive immediate insights into their operations. Whether tracking social media sentiment, analyzing IoT sensor data, or making real-time financial decisions, DataGalaxy's data lake provides the query performance needed for these critical applications.
DataGalaxy also caters to historical data analysis. Data professionals can delve into archived data to identify trends, patterns, and historical insights. This capability is invaluable for healthcare, finance, and retail industries, where historical data analysis is essential for strategic planning and compliance.
Data lakes for robust data governance
Data governance involves defining and enforcing policies, procedures, and standards to ensure data quality, compliance, and accountability. This ensures that data ownership is clearly defined and quality maintained throughout its lifecycle.
Data lakes also support metadata management, which is essential for data governance. Metadata provides valuable information about data assets, including their lineage, source, and usage. This transparency enhances data understanding and accountability, ensuring organizations can trust their data.
Conclusion
In conclusion, understanding the differences between data lakes and data warehouses is vital for organizations seeking to optimize their data strategies. Data lakes offer unparalleled flexibility, storing raw and unstructured data in its original form, making them ideal for handling diverse data types and enabling real-time analytics. This adaptability is essential for industries that require quick responses to changing data needs.
On the other hand, data warehouses provide a highly structured environment where data is meticulously organized and transformed before storage. This ensures data consistency, integrity, and efficiency, making it perfect for industries with stringent data regulations and the need for fast, reliable querying.
While data lakes excel in scalability and handling vast volumes of unstructured data, data warehouses are indispensable for organizations that prioritize data accuracy and structured analysis. By leveraging the strengths of both systems, organizations can create a comprehensive data management strategy that meets their unique needs.