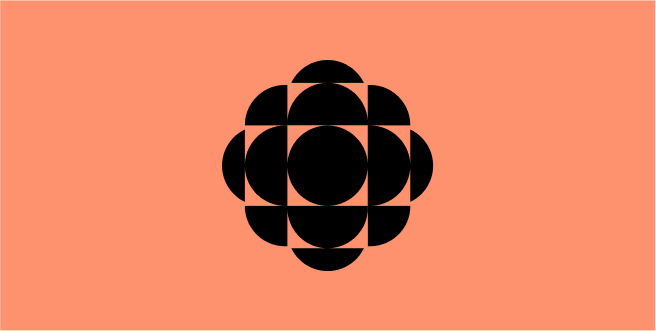
Data mesh vs. data lake: What’s the difference?
While many are familiar with the concept of data lakes, the emergence of the data mesh paradigm has led to inevitable comparisons between the two. So, when discussing data mesh and data lake, what exactly sets them apart?
Defining data lake & data mesh
Before delving into their differences, it is crucial to understand what each term represents.
- Data lake: A data lake is a centralized repository that can store vast amounts of structured and unstructured data in its raw format. It is akin to a vast reservoir, pooling data from various sources and making it accessible for diverse analytical and processing tasks.
- Data mesh: Going beyond the centralized model, a data mesh approaches data as a decentralized product - Instead of one centralized repository, data is distributed across multiple domains or teams. Each domain is responsible for its data product, fostering ownership and domain-specific optimizations. For many, understanding data mesh translates to seeing data as a product, decentralized, and domain-oriented.
Key differentiators between data mesh & data lakes
- Centralized vs. decentralized approach: As discussed, a primary distinction lies in their architectural approach: While a data lake aggregates data in a single, centralized repository, a data mesh distributes it across multiple domain-oriented sources.
- Ownership & responsibility: In a data lake model, ownership often rests with a central IT or data team. Conversely, the data mesh model distributes ownership, with each domain or team taking full responsibility for its data from source to provision.
- Scalability & flexibility: While data lakes can handle vast volumes of data, their centralized nature might introduce scalability challenges. Data mesh, being decentralized, can offer more flexibility and scalability, as each domain can scale its infrastructure independently.
- Data quality & governance: In the data lake model, governance and quality control measures are applied centrally. In contrast, with the data mesh paradigm, as each domain owns its data, there is an inherent responsibility to maintain its quality, fostering a sense of accountability.
- Tooling & infrastructure: Data lakes often rely on specific tooling and infrastructure optimized for large-scale, centralized storage and processing. On the other hand, in a data mesh environment, each domain might employ tools best suited to its specific needs, leading to a more diverse technological landscape.
- Data integration & ingestion: In a data lake, integrating data from diverse sources into the centralized repository can be complex. It requires consistent ETL (Extract, Transform, Load) processes. In the case of a data mesh, data is integrated at the domain level, potentially allowing for more tailored integration techniques.
- The granularity of data access: With a centralized structure like the data lake, granular access controls might be challenging. In a decentralized structure like the data mesh, it is easier to define granular access at the domain or product level, aligning with specific business rules.
- Cost implications: The centralized nature of data lakes often means a significant upfront investment in infrastructure and storage. Data meshes, with their distributed setup, might allow organizations to optimize costs based on domain-specific needs.
- Agility in data operations: Data mesh's decentralized model can lead to increased agility. With domains or teams managing their data, iterations or changes can be faster without the bureaucracy that sometimes comes with centralized models like the data lake.
- Search & discovery: In data lakes, as data accumulates, search and discovery can become challenging. While data catalogs can mitigate this, it is a hurdle. In a data mesh, the decentralized nature means smaller, domain-specific datasets, potentially making discovery more intuitive.
- Maintenance & operations: The upkeep of a centralized system like a data lake requires dedicated resources. Operational issues can affect the entire system. In a data mesh, issues might be isolated to individual domains, reducing the risk of widespread disruption.
- Adherence to regulations: Regulatory compliance, especially regarding data, is crucial. Data lakes, due to their centralized nature, may have a singular compliance framework. A data mesh, however, may allow domains to adhere to regulations specific to their function or geography.
- Data evolution & versioning: As data evolves, versioning becomes essential. In a data lake, managing versions of vast datasets can be intricate. With data mesh, the decentralized approach means each domain can have its versioning system tailored to its needs, potentially simplifying data evolution tracking.
The role of metadata & data catalogs in data mesh and data lakes
In both models, understanding the data's origins, relationships, and transformations is pivotal.
This is where data lineage and data catalogs come into play. For data lakes, with their vast reservoirs of data, using a data catalog can help users navigate and find relevant datasets. It provides metadata, descriptions, and more, ensuring that data consumers can trust and understand the data they are accessing.
In a data mesh setup, given the decentralized nature, the role of metadata becomes even more crucial. Here, a data catalog not only aids in data discovery but also ensures that there's consistency in how data is described and accessed across domains.
Data mesh vs. data lake: Use cases
Understanding the practical applications of each can further clarify the data lake vs. data mesh debate:
- Data lake:
- Large enterprises looking for a centralized data repository to serve diverse analytical needs.
- Organizations aiming to store vast volumes of raw data cost-effectively for future processing and analytics.
- Data mesh:
- Companies with multiple teams or domains wishing to maintain autonomy over their data while ensuring its availability enterprise-wide.
- Organizations looking to foster a culture of data ownership and domain-specific optimizations.
Data lake vs. data mesh for your teams
Data mesh vs. data lake is not necessarily a debate about superiority.
Instead, it is about understanding organizational needs, the volume and diversity of data handled, and the desired level of decentralization. While data lakes offer a proven model for centralized data storage and analytics, data mesh introduces a paradigm shift, emphasizing domain ownership and decentralized governance.
Conclusion
As organizations continue to evolve, their choice between these models will be influenced by their specific data challenges and objectives. What remains constant, however, is the need for robust metadata management, data quality, and governance practices, ensuring that data remains an invaluable asset driving insights and innovations.