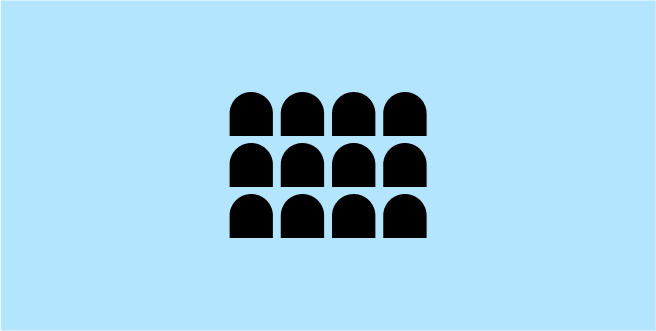
How to create & sustain a data quality management process
Your business runs on data. But how reliable is that data?
If you're making decisions based on questionable quality data, you should question the results. The risks are even higher for AI-first companies. AI doesn't fix bad data; it recycles it.
You need a data quality management (DQM) process to deliver trusted, business-ready data at scale. So, what makes a great data quality management process tick?
What's in a data quality management process?
A first-rate data quality management (DQM) process rests on a core set of elements and a proven process to keep data accurate, consistent, and business-ready - The definition of data quality.
Let's break down the key components and how they work to make DQM not just another back-end process but a competitive advantage.
1. Determine what "Good" looks like
There isn't a universal standard for data quality. It varies from one company to the next. Work with your organization's stakeholders to determine the level of quality needed to support their use and operation of data and set a standard that supports them.
2. Profile data at the source
Data profiling spots errors, missing fields, anomalies, and outliers as data is ingested. Catching minor errors early is key to avoiding larger problems later, and it represents the first line of defense against poor-quality issues.
3. Cleaning & standardizing
Data cleansing corrects, standardizes, and enriches your data. Pro tip: Use automated tools to streamline and speed cleansing to keep your AI pipelines sparkling clean and noise-free.
4. Validate at every source
Build validation rules into every data ingestion point to enforce your pre-defined standards at every junction. This is your second line of defense against low-quality data.
5. Create accountability for data governance
Move beyond simply setting standards by tasking people to uphold them. Appoint data owners and stewards to champion and lead your data governance and quality efforts.
Also, use role-based controls and automated workflows to bake your standards directly into your workflows.
6. Continuous monitoring & improvement
Things change, so quality standards need continual reevaluation and refinement. Use dashboards, trend analysis, and automated alerts to track data health and emerging issues.
Make quality audits, root-cause reviews, and stakeholder feedback a routine part of your data lifecycle—not an annual fire drill.
How DataGalaxy supports data quality management
A solid data quality management strategy is only as good as the tools behind it. Without data observability and automation, it's nearly impossible to enforce quality, monitor trends, or fix problems.
DataGalaxy's data quality monitoring is purpose-built to help you move from reactive data firefighting to proactive quality control from inside your data ecosystem.
Here's how DataGalaxy supports your DQM process every step of the way:
With DataGalaxy, data quality management stops being a siloed back-office function and becomes a collaborative, always-on part of your operational rhythm. That's how you scale trust—in your data and your decisions.
Your blueprint for data quality
Creating and sustaining a high-impact data quality management process means turning best practices into everyday habits.
Start by defining your quality standards and aligning them with real business needs. Then, embed those standards into your data pipelines with profiling, validation, and cleansing at the source. Build a culture of accountability with clear ownership and support it with tools that enable continuous monitoring, alerting, and collaboration.
With DataGalaxy, these building blocks come together in a connected, automated ecosystem. They help you move from reactive fixes to proactive quality assurance. When data quality is built into how you work, trusted data becomes your default - Not your exception.