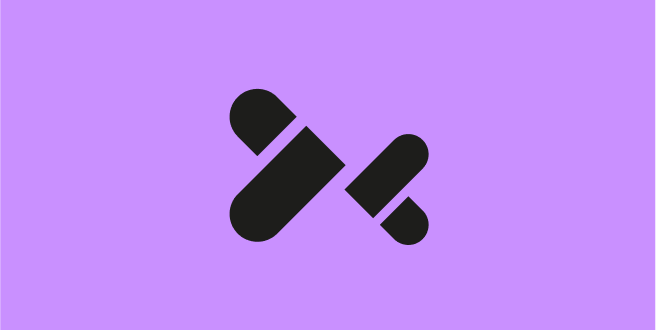
The increasing need for data trust: 2 real-world examples
Artificial intelligence is becoming increasingly crucial for businesses. However, to fully leverage AI's potential, organizations must ensure data readiness and data trust.
This involves implementing robust data governance and data observability strategies.
This article explores how these strategies can pave the way for AI readiness, drawing insights from a recent presentation on the topic.
Data governance vs. data observability
Let’s get the basics first: Building AI readiness and trust requires a strong understanding of the role data governance and data observability play in unlocking its full potential.
By definition, data governance is essential for helping people distinguish between reliable and unreliable data. For example, it prevents analytics teams from consuming unreliable or unauthorized data.
Data observability, on the other hand, ensures that data is healthy and fresh at usage time for all users. It helps identify areas where data quality is poor and supports data stewards with remediation options.
The data trust challenge
For data management professionals, data trust refers to the confidence that an organization and its stakeholders can place in the integrity, accuracy, and reliability of its data assets.
It is the assurance that data is consistently high-quality, well-governed, secure, and used ethically throughout its lifecycle.
Building data trust involves implementing robust data governance frameworks, clearly defining data ownership and stewardship roles, and ensuring traceability through the use of metadata and data lineage. It also means enforcing policies around data privacy, access control, and compliance to minimize risk and support responsible data use.
In practical terms, data trust is a foundational enabler of digital transformation, advanced analytics, and AI initiatives. When teams trust their data, they can make faster, more informed decisions, collaborate more effectively, and drive innovation with confidence.
Conversely, a lack of data trust leads to duplicated efforts, poor decision-making, and a hesitancy to embrace data-driven initiatives. For professionals in data management, fostering data trust is not a one-time task - It’s an ongoing strategy that aligns technology, processes, and culture to treat data as a valued asset.
The real-world impact of data governance
Society Insurance
Challenge:
As a rapidly growing insurance provider, Society Insurance faced several data governance challenges.
Its decentralized approach to data management, reliance on Excel and SharePoint, and lack of clear data ownership hindered the company’s ability to manage its expanding data ecosystem effectively.
With an increasing number of data sources and an evolving infrastructure, the need for a unified, scalable solution became critical. Without a comprehensive governance framework, the company struggled with fragmented data definitions, siloed information, and inefficient collaboration between business and IT teams.
Solution:
Society Insurance adopted a people-first approach to data governance, focusing on enhancing data literacy and promoting collaboration across the organization.
The company implemented DataGalaxy, a centralized platform that provided a unified view of its data assets, standardized metadata definitions, and ensured transparency in data lineage.
This platform empowered employees, from business to IT, to actively engage with data, driving adoption and improving decision-making across departments.
Implementation:
The implementation of DataGalaxy was completed in just 10 months, from business case approval to company-wide deployment.
By integrating tools like DataGalaxy’s glossary and dictionary, Society Insurance created a clear framework for data ownership and responsibilities, improving accountability. Additionally, business teams could now align their operations with standardized data definitions, bridging the gap between IT and business functions.
Kirsten Kerr, Manager of Data and Data Governance at Society Insurance, commented,
"The biggest feature is… just simply the connection between the glossary and the dictionary. It enables IT to understand business definitions and how they’re applied in the Enterprise Data Warehouse (EDW).”
Results:
The move to DataGalaxy significantly improved operational efficiency and decision-making across Society Insurance. Key outcomes include:
Enhanced risk management
The improved ability to investigate claim causes and develop prevention strategies led to better risk mitigation and policyholder experiences.
Boosted data literacy
Empowering employees across the organization to engage with and understand data enhanced informed decision-making, aligning departments around a common data language.
Increased operational efficiency
The streamlined process for creating data reports and quality checks accelerated the production of data-driven insights, reducing time-to-value.
By bridging the gap between IT and business, Society Insurance is not only fostering a culture of data literacy but also ensuring regulatory compliance and transparency in data usage.
Business Impact:
Society Insurance’s adoption of DataGalaxy has resulted in tangible business value, including:
Improved data governance
By establishing clear ownership and documentation for data assets, the company has created a solid foundation for future scalability and regulatory compliance.
Faster decision-making
With all employees speaking the same data language, the company has reduced inconsistencies and confusion in data interpretation, leading to better business decisions.
Compliance & risk mitigation
Enhanced ability to classify sensitive data and meet audit requirements has strengthened Society Insurance’s position in a highly regulated industry.
Through its people-first approach, Society Insurance has set a new standard for how organizations can leverage data governance platforms to empower employees, improve collaboration, and drive business value.
The real-world impact of data observability
Zoom
Challenge:
As Zoom experienced rapid growth, it faced challenges with managing fragmented data quality.
The company’s reliance on manual SQL checks for monitoring data quality became unsustainable. This process often led to prolonged resolution times, which negatively impacted workflows and caused delays in addressing data issues.
With Zoom’s expanding data operations, it became clear that a more scalable, automated solution was needed to manage and maintain data integrity.
Solution:
To tackle this challenge, Zoom adopted Bigeye, a data observability tool designed to automate data quality monitoring.
By integrating data observability into their data product workflows, Zoom was able to monitor data health and quickly identify issues proactively.
With this new approach, the team can now detect 2-3 potential data issues each month before they are flagged by the business, allowing for a more efficient and effective resolution process.
Implementation:
Zoom’s data team leveraged Bigeye’s REST API and Airflow operator to monitor the success of their ingestion jobs, ensuring seamless data pipeline execution.
Meanwhile, business teams implemented Bigeye’s YAML-based monitoring, which automated data quality checks across the board. This combination of tools created a proactive data monitoring framework that alerts the team to any anomalies in real time.
Tina Chang, a Data Engineer at Zoom, shared the impact:
“Now, I get immediate insights when something is wrong, so I can proactively notify downstream users and correct it before it causes a problem.”
Results:
With automated data observability in place, Zoom has streamlined its data quality management.
This solution not only reduces the risk of data issues disrupting operations but also empowers the team to take swift action before problems escalate.
By implementing Bigeye, Zoom is improving overall data health, driving higher productivity, and ensuring that data quality no longer becomes a bottleneck to business success.